금요일! 😎
어제 잠깐 소개한 Logistic Regression을 배운다~
Linear Regression(연속적인 숫자 값 예측)이 발전한 것이 Logistic Regression → Classification(분류를 판단하는 예측)
- Binary Classification(이항분류)
- Multinomial Classification(다항분류)
그래프를 볼 수 있는 유틸리티 모듈(mglearn)을 추가적으로 설치하자
conda activate maching_TF15
pip install mglearn
conda install은 이미 설치되어 있는 모듈, 패키지에 대한 Dependency를 고려해서 최적인 버전을 설치,
pip install은 그냥 깔아버림
Logistic Regression : Linear Regression을 이용해 Training Data Set의 특성 · 분포를 가장 잘 표현하는 선을 찾고, 이를 기준으로 0과 1로 분류하는 알고리즘. 정확도가 상당함. Deep Learing(Neural Network)의 기본 component로 사용함
시그모이드 함수를 이용해 0과 1로 분류
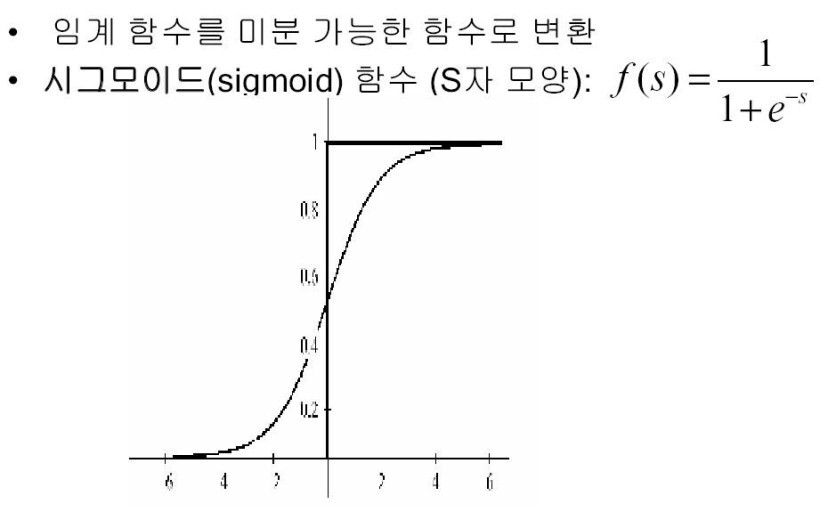
1. Logistic Regression
import numpy as np
from sklearn import linear_model
import mglearn # utility module. data set을 가지고 있음
import matplotlib.pyplot as plt
import warnings
warnings.filterwarnings(action='ignore')
# Training Data Set
x,y = mglearn.datasets.make_forge()
# print(x) # 독립변수(2차원 ndarray)
# print(y) # 종속변수(1차원 ndarray)
mglearn.discrete_scatter(x[:,0], x[:,1], y)
plt.legend(['class 0', 'class 1'], loc='best')
# plt.show()
model = linear_model.LinearRegression()
model.fit(x[:,0].reshape(-1,1), x[:,1].reshape(-1,1))
plt.plot(x[:,0], x[:,0]*model.coef_.ravel() + model.intercept_, color='r')
plt.show()
2. 분류 문제를 Logistic Regression가 아닌 Linear Regression으로 해결할 수 있을까?
지대값(독립변수의 이상치)이 들어올 경우 예측 error
import numpy as np
from sklearn import linear_model
import matplotlib.pyplot as plt
x_data = np.array([1, 2, 5, 8, 10, 30]) # 독립변수. 공부시간
t_data = np.array([0, 0, 0, 1, 1, 1]) # 종속변수. 합격여부(0 : 불합격, 1 : 합격)
# model 생성
model = linear_model.LinearRegression()
# model 학습
model.fit(x_data.reshape(-1,1), t_data.reshape(-1,1))
# prediction
print(model.predict(np.array([[7]]))) # [[0.41831972]]. 0.5보다 크다! 아까는 붙었는데 왜 불합격?!
plt.scatter(x_data, t_data)
plt.plot(x_data, x_data*model.coef_.ravel() + model.intercept_)
plt.show()
3. Cross-Entropy : loss func의 형태가 볼록 함수(convex func)가 아닐 경우
경사하강법에 의해 local minima에서 진동하므로, global minima를 찾을 수 없음
→ Logistic Regression에 대한 loss func 수식 변형함(Cross-Entropy 혹은 log loss)
4. Logistic Regression(Binary Classification)
5. Logistic Regression(Binary Classification) 구현 - Python
import numpy as np
# 다변수 함수의 수치미분 코드
def numerical_derivative(f,x):
delta_x = 1e-4
derivative_x = np.zeros_like(x)
it = np.nditer(x, flags=['multi_index'])
while not it.finished:
idx = it.multi_index
tmp = x[idx]
x[idx] = tmp + delta_x
fx_plus_delta = f(x)
x[idx] = tmp - delta_x
fx_minus_delta = f(x)
derivative_x[idx] = (fx_plus_delta - fx_minus_delta) / (2 * delta_x)
x[idx] = tmp
it.iternext()
return derivative_x
# Training Data Set
x_data = np.array([2,4,6,8,10,12,14,16,18,20]).reshape(-1,1) # 행렬곱연산을 위해 2차원으로 reshape
t_data = np.array([0,0,0,0,0,0,1,1,1,1]).reshape(-1,1)
# Weight, bias 정의
W = np.random.rand(1,1)
b = np.random.rand(1)
# Logistic Regression Model, Predict Model, Hypothesis
def predict(x):
z = np.dot(x,W) + b # Linear Regression Model. np.dot은 행렬곱연산
y = 1 / (1 + np.exp(-1 * z)) # Logistic Regression Model
result = 0 # 계산되는 값은 결국 0 ~ 1 사이
if y >= 0.5:
result = 1
else:
result = 0
return y, result
# Cross Entropy(log loss)
def loss_func(input_data): # [W b]
input_W = input_data[:-1].reshape(-1,1)
input_b = input_data[-1]
z = np.dot(x_data,input_W) + input_b
y = 1 / (1 + np.exp(-1 * z))
delta = 1e-7
# delta로 아주 작은 값을 부여하기 때문에 0이 나와서 오류나지 않음
return - 1 * np.sum(t_data * np.log(y + delta) + (1 - t_data) * np.log(1 - y + delta))
# learning rate
learning_rate = 1e-4
# 반복학습
for step in range(300000):
input_param = np.concatenate((W.ravel(), b.ravel()), axis=0)
derivative_result = learning_rate * numerical_derivative(loss_func, input_param)
W = W - derivative_result[:-1].reshape(-1,1)
b = b - derivative_result[-1]
if step % 30000 == 0:
input_param = np.concatenate((W.ravel(), b.ravel()), axis=0)
print('W : {}, b : {}, loss : {}'.format(W, b, loss_func(input_param)))
# Prediction
study_hour = np.array([[13]]) # 12시간은 불합격, 14시간은 합격
y_prob, result = predict(study_hour)
print('합격확률 : {}, 합격여부 : {}'.format(y_prob,result)) # 합격확률 : [[0.54440637]], 합격여부 : 1
6. Logistic Regression(Binary Classification) 구현 - Sklearn
from sklearn import linear_model
# Training Data Set
x_data = np.array([2,4,6,8,10,12,14,16,18,20]).reshape(-1,1) # 행렬곱연산을 위해 2차원으로 reshape
t_data = np.array([0,0,0,0,0,0,1,1,1,1]).reshape(-1,1)
# Model 생성
model = linear_model.LogisticRegression()
# Model 학습
model.fit(x_data, t_data)
# Prediction
study_hour = np.array([[13]])
result = model.predict(study_hour) # 확률을 제외한 합격여부만 알려줌
result_prob = model.predict_proba(study_hour) # 불합격 / 합격 확률
print('합격확률 : {}, 합격여부 : {}'.format(result_prob,result)) # 합격확률 : [[0.50009391 0.49990609]], 합격여부 : [0]?!
7. Logistic Regression(Binary Classification) 구현 - Tensorflow
import tensorflow as tf
import warnings
warnings.filterwarnings('ignore')
# Training Data Set
x_data = np.array([2,4,6,8,10,12,14,16,18,20]).reshape(-1,1)
t_data = np.array([0,0,0,0,0,0,1,1,1,1]).reshape(-1,1)
# Placeholder
X = tf.placeholder(shape=[None, 1], dtype=tf.float32)
T = tf.placeholder(shape=[None, 1], dtype=tf.float32)
# Weight, bias
W = tf.Variable(tf.random.normal([1,1]))
b = tf.Variable(tf.random.normal([1]))
# Model 생성
logit = tf.matmul(X,W) + b # Linear Regression Model
H = tf.sigmoid(logit) # Logistic Regression Model. Sigmoid func
# loss function
loss = tf.reduce_mean(tf.nn.sigmoid_cross_entropy_with_logits(logits=logit, labels=T)) # 인자 두 개
# train node
train = tf.train.GradientDescentOptimizer(learning_rate=1e-3).minimize(loss)
# Session & 초기화
sess = tf.Session()
sess.run(tf.global_variables_initializer())
# 반복 학습
for step in range(30000):
_, W_val, b_val, loss_val = sess.run([train, W, b, loss], feed_dict={X: x_data, T: t_data})
if step % 3000 == 0:
print('W : {}, b: {}, loss : {}'.format(W_val, b_val, loss_val))
# Prediction
study_hour = np.array([[13]])
result = sess.run(H, feed_dict={X: study_hour})
print('합격확률 : {}'.format(result)) # 합격확률 : [[0.5792821]]
이렇게 Logistic Regression을 Python, Sklearn, Tensorflow 3가지로 구현했다.
이 중 Sklearn이 정답에 가깝기 때문에 지금까지는 다른 모델들의 예측치를 Sklearn과 비교했다.
실제 그 값이 맞는지 확인은 다음 주에 배울 평가지표(Metrics)로 진행~
오늘도 실습 과제가 있다. 대학원 데이터셋으로 위의 3가지 방법을 사용해 Logistic Regression 구현하기!
1. 3/15 화 | 2. 3/16 수 | 3. 3/17 목 | 4. 3/18 금 | 5. 3/21 월 |
ML 환경 설정 (아나콘다, 주피터 노트북), Pandas, Numpy, ndarray |
ML Numpy, 행렬곱연산, 전치행렬, iterator, axis, Pandas, Series, DataFrame |
ML Pandas, DataFrame, 함수들 |
ML Pandas, 데이터 분석, 데이터 전처리 |
ML Pandas, 데이터 전처리, 수행평가 |
6. 3/22 화 | 7. 3/23 수 | 8. 3/24 목 | 9. 3/25 금 | 10. 3/28 월 |
ML 데이터 시각화, Matplotlib, 기술통계 |
ML 기술통계 |
ML 기술통계, 머신러닝 |
ML 미분, Regression |
ML 머신러닝 기법들, Regression |
11. 3/29 화 | 12. 3/30 수 | 13. 3/31 목 | 14. 4/1 금 | 15. 4/4 월 |
ML Regression 구현 (Python, Scikit-Learn) |
ML Outlier, Z-score, MinMaxScaler, 다변수 |
ML Tensorflow, Classification |
ML Classification, Logistic Regression |
ML 평가지표(Metrics) |
* 수식과 흐름을 머리에 넣어놓고 돌아가는 구조를 상기하자!